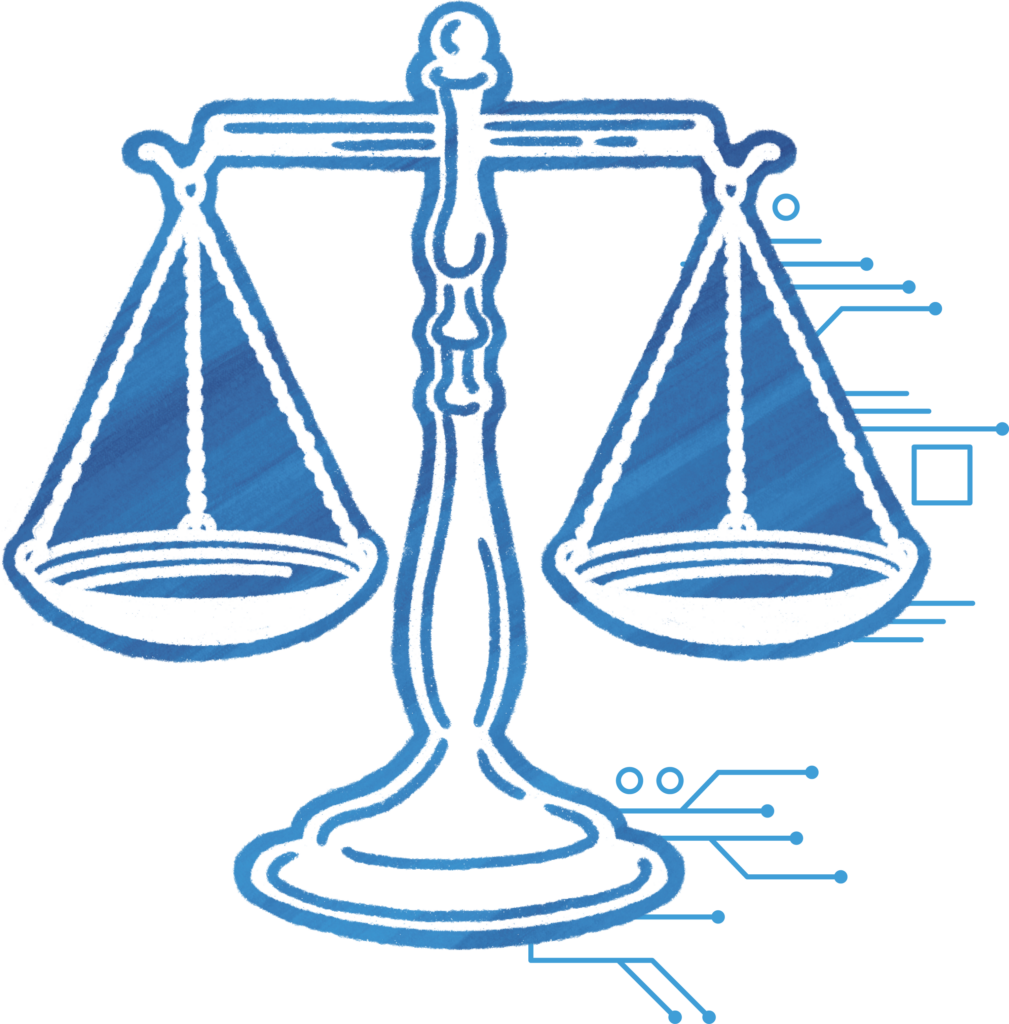
1
Accountability: Keeping clear data records tracking the timeline and responsibilities of key decisions to prevent retrospective ‘ethics washing’ practices.
2
Transparency: Make these data records easily accessible, including disclosing how generative AI has been used in any production process at the point of media release.
3
Redress bias: Use of generative AI tools with an active awareness that generative AI models can produce biased, stereotyped and sometimes harmful outputs, as they are replicating underlying bias within the datasets on which they are trained. Redress this by embedding intersectionality and cultural specificity into your prompts as much as possible.
4
Collaboration: Recognise the need for both human and machine labour in production processes, including the material financial consequences for human creatives.
5
Interdisciplinarity: Ensure diversity of stakeholders in the production process, both in terms of cultural identity and technical background, to mitigate potential bias and prevent homogenised outputs.
6
Informed participation: Ensure that the use of any stakeholder data, including images and voices that can be manipulated digitally with AI, falls clearly within the original terms as set out in any contract or agreement. Consent should be informed, affirmative and opt-in, rather than opt-out. Ensure legal compliance with data protection, privacy, copyright and Intellectual Property laws when handling this data.
7
Open datasets (where applicable): For those with the technical ability, training and access to do so, develop and use your own localised model, trained on data you already have the copyright for, that adheres to the principle of informed consent (and fair remuneration where appropriate) for artists whose work you may be using in the training process. Make this dataset open and transparent to the public upon release of any finished artefacts. For users who do not have the means to do this, further training and education are required to help people transition toward the development of their own models.